Unleash the Power of Qwen3: The Fantastic Open-Source AI Model Outperforming Rivals
Unleash the Power of Qwen3: Outperform Rivals with this Remarkable Open-Source AI Model. Discover Qwen3's impressive benchmarks, hybrid reasoning capabilities, and seamless integration with Zapier's MCP tools. Optimize your AI workflows for efficiency and performance.
2025年6月29日
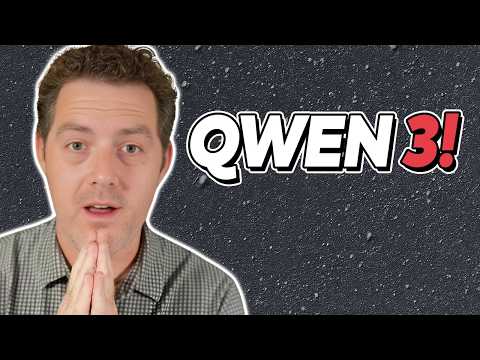
Qwen3 is a powerful open-source AI model that delivers exceptional performance across a wide range of tasks, from coding and scientific reasoning to general language understanding. This blog post explores the model's impressive capabilities, including its hybrid thinking approach, optimized MCP tool integration, and state-of-the-art benchmarks that outshine even the latest Llama 4 model. Discover how Qwen3's innovative architecture and extensive pre-training make it a game-changer in the world of large language models.
Quen 3 Models: The Flagship and the Efficient Performers
Hybrid Thinking Capability: The Key Differentiator
Model Architecture and Performance Benchmarks
Data Collection and Pre-Training Process
Post-Training Pipeline: Developing the Hybrid Model
Availability and Comparison to Llama 4
Conclusion
Quen 3 Models: The Flagship and the Efficient Performers
Quen 3 Models: The Flagship and the Efficient Performers
The Quen 3 model family offers a range of powerful and efficient AI models, catering to diverse needs. The flagship Quen 3 235B model boasts an impressive 235 billion parameters, with 22 billion active parameters. This model showcases impressive performance across a variety of benchmarks, including Arena Hard, Amy 24/25, Live Codebench, and BFCL.
Alongside the flagship model, Quen 3 also introduces a highly efficient 30 billion parameter model with just 3 billion active parameters. This compact model is designed to be lightning-fast on user machines, making it an excellent choice for applications that prioritize speed and efficiency.
The Quen 3 family further includes a range of dense models, ranging from 32 billion to 600 million parameters. These models offer a balance of performance and resource requirements, catering to a wide range of use cases.
A standout feature of the Quen 3 models is their hybrid approach to problem-solving. These models can operate in both a "thinking mode" and a "non-thinking mode," allowing users to control the level of reasoning and depth of analysis based on the task at hand. This flexibility enables more optimal performance and resource utilization, making Quen 3 a versatile choice for a variety of applications, including coding, task automation, and complex problem-solving.
Hybrid Thinking Capability: The Key Differentiator
Hybrid Thinking Capability: The Key Differentiator
The Quen 3 models introduce a unique hybrid approach to problem-solving, offering both a "thinking mode" and a "non-thinking mode". In the thinking mode, the model takes time to reason step-by-step before delivering the final answer, making it ideal for complex problems that require deeper thought. In the non-thinking mode, the model provides quick, near-instant responses, suitable for simpler questions where speed is more important than depth.
This flexibility allows users to control the model's thinking budget based on the task at hand, achieving a more optimal balance between cost-efficiency and inference quality. For tasks like code generation or complex problem-solving, users can allocate a higher thinking budget, while for simpler tasks like running terminal commands, the non-thinking mode can be leveraged for faster responses.
The integration of these two modes greatly enhances the model's ability to implement stable and efficient thinking budget control, making it a powerful tool for a wide range of applications, from coding and task automation to general problem-solving.
Model Architecture and Performance Benchmarks
Model Architecture and Performance Benchmarks
The Quen 3 model family consists of a range of architectures, including two Mixture of Experts (MoE) models and six dense, more traditional models. The flagship Quen 3 235B model has 235 billion parameters, with 22 billion active parameters and 128 experts, of which 8 are activated at inference time. The context length for this model is 128k tokens, which is the current industry standard.
The Quen 3 30B model is a highly efficient MoE model, with only 3 billion active parameters out of a total of 30 billion. This makes it an excellent choice for deployment on consumer hardware, as it can be easily accommodated on GPUs. This model has 48 layers, 128 total experts, and an activation of 8 experts at inference time, with a 128k token context length.
The dense models in the Quen 3 family range from 32B down to 600M parameters, with the smaller models having a 32k token context length, while the larger ones maintain the 128k context length. One of the standout features of these dense models is their ability to perform tool calling during the chain of thought process, allowing them to execute tasks such as fetching GitHub stars and plotting a bar chart, as well as organizing a desktop by file type.
The pre-training process for Quen 3 was significantly expanded compared to Quen 2.5, with the model being trained on nearly twice the amount of data, 36 trillion tokens, across 119 languages and dialects. The data sources included not only web content but also PDF-like documents, with the previous generation of models (Quen 2.5VL and Quen 2.5) used to extract and improve the quality of the content.
The pre-training process consisted of three stages: the first stage provided the model with basic language skills and general knowledge, the second stage increased the proportion of knowledge-intensive data such as STEM, coding, and reasoning tasks, and the final stage extended the context length to 32k tokens.
The development of the hybrid model, capable of both step-by-step reasoning and rapid responses, involved a four-stage training pipeline. This included long chain of thought training, reasoning reinforcement learning, thinking model fusion, and general reinforcement learning across multiple domains.
The performance of the Quen 3 models is impressive, with the flagship 235B model outperforming the Llama 4 Maverick model across a range of benchmarks, including MMLU, SuperGPQA, and GSMA. The smaller 30B model also demonstrates excellent performance, particularly in the GPQA Diamond Scientific Reasoning benchmark, where it outperforms larger models like Llama 4 Maverick.
Data Collection and Pre-Training Process
Data Collection and Pre-Training Process
The Quen 3 models have been significantly expanded compared to the previous Quen 2.5 generation. The pre-training dataset has nearly doubled, using 36 trillion tokens across 119 languages and dialects.
The data collection process involved not only web-crawled data, but also PDF-like documents. The team used the previous Quen 2.5VL model to extract text from these documents, and the Quen 2.5 model to improve the quality of the extracted content.
To increase the amount of math and code data, the team used the Quen 2.5 math and Quen 2.5 coder models to generate synthetic data, including textbooks, question-answer pairs, and code snippets.
The pre-training process had three stages:
-
Stage 1: The model was pre-trained on over 30 trillion tokens with a context length of 4,000 tokens. This stage provided the model with basic language skills and general knowledge.
-
Stage 2: The data set was improved by increasing the proportion of knowledge-intensive data, such as STEM, coding, and reasoning tasks. The model was then pre-trained on an additional 5 trillion tokens.
-
Stage 3: High-quality, long-context data was used to extend the context length to 32,000 tokens.
The post-training process was also crucial in developing the hybrid model capable of both step-by-step reasoning and rapid responses. This involved a four-stage training pipeline:
-
Long Chain of Thought: The base model was post-trained on long chain of thought data covering various tasks and domains, such as mathematics, coding, logical reasoning, and STEM problems.
-
Reasoning Reinforcement Learning: The focus was on scaling up computational resources for reinforcement learning, utilizing rule-based rewards to enhance the model's exploration and exploitation capabilities.
-
Thinking Model Fusion: Non-thinking capabilities were integrated into the model by fine-tuning it on a combination of long chain of thought data and commonly used instruction tuning data.
-
General Reinforcement Learning: Reinforcement learning was applied across more than 20 general domain tasks to further strengthen the model's general capabilities and correct undesired behaviors.
Finally, the team used strong token distillation to create the smaller versions of the Quen 3 models, making them available for download on platforms like LM Studio and O Llama within MLX, Llama CPP, and K Transformers.
Post-Training Pipeline: Developing the Hybrid Model
Post-Training Pipeline: Developing the Hybrid Model
To develop the hybrid model capable of both step-by-step reasoning and rapid responses, the Quen 3 team implemented a four-stage training pipeline:
-
Long Chain of Thought: In the first stage, they used long chain of thought data covering various tasks and domains such as mathematics, coding, logical reasoning, and STEM problems. This was aimed at equipping the model with fundamental reasoning abilities.
-
Reasoning Reinforcement Learning: The second stage focused on scaling up computational resources for reinforcement learning, utilizing rule-based rewards to enhance the model's exploration and exploitation capabilities.
-
Thinking Model Fusion: In the third stage, they integrated non-thinking capabilities into the model by fine-tuning it on a combination of long chain of thought data and commonly used instruction tuning data. The data was generated by the enhanced thinking model from the second stage, ensuring a seamless blend of reasoning and quick response capabilities.
-
General Reinforcement Learning: In the fourth stage, they applied reinforcement learning across more than 20 general domain tasks to further strengthen the model's general capabilities and correct undesired behaviors.
This multi-stage training pipeline allowed the Quen 3 team to develop a hybrid model that can effectively balance step-by-step reasoning and rapid responses, depending on the task at hand. The integration of these two modes of operation greatly enhances the model's ability to implement stable and efficient thinking budget control, enabling users to configure task-specific budgets with greater ease and achieve a more optimal balance between cost-efficiency and inference quality.
Availability and Comparison to Llama 4
Availability and Comparison to Llama 4
Quen 3 is now available for download on LM Studio as well as O Lama within MLX, Llama CPP, and K Transformers. This open-source and open-weights model is comparable to Gemini 2.5 Pro and the recently launched Quen 3 model.
When compared to Llama 4 Maverick, Quen 3's flagship 235B model outperforms it across various benchmarks. In MMLU, Quen 3 scored 87% compared to Llama 4's 85%. Similarly, in GPQA, Quen 3 achieved 44% while Llama 4 scored 40%. The model also showed improvements in other tasks such as GSM8K.
Independent benchmarks conducted by Artificial Analysis further highlight Quen 3's capabilities. In the GPQA Diamond Scientific Reasoning test, Quen 3's 235B model scored 70%, placing it behind Gemini 2.5 Pro at 84% but ahead of Deepseek R1 and Llama 3.1 Neatron Ultra.
Interestingly, the 30B Quen 3 model with only 3B active parameters performs exceptionally well on the GPQA Diamond benchmark, showcasing its efficiency and reasoning abilities.
Overall, Quen 3 presents a compelling open-source alternative to Llama 4, offering impressive performance and the flexibility of hybrid thinking and non-thinking modes.
Conclusion
Conclusion
The Quen 3 models represent a significant advancement in large language models, offering impressive performance across a range of benchmarks. The hybrid approach, which allows for both quick responses and deeper reasoning, is a particularly noteworthy feature that enhances the model's versatility and usefulness.
The extensive pre-training process, which leverages previous generation models and synthetic data, has resulted in models with strong language skills, general knowledge, and specialized capabilities in areas like mathematics, coding, and logical reasoning.
The availability of multiple model sizes, from 600 million to 235 billion parameters, provides users with the flexibility to choose the most appropriate model for their specific needs, balancing performance and computational requirements.
The integration of MCP (Model Calling Protocol) tools, facilitated by the partnership with Zapier, further expands the capabilities of the Quen 3 models, allowing for seamless integration with a wide range of applications and workflows.
Overall, the Quen 3 models demonstrate the continued progress in the field of large language models, offering a compelling alternative to existing solutions and showcasing the potential for even more advanced AI systems in the future.
常問問題
常問問題